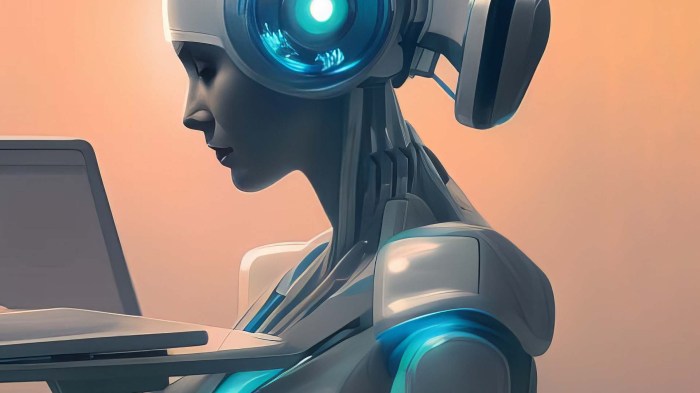
Keep Pace with AI Developments: Intermediate Level Course 30
Keep pace with developments ai with intermediate level course 30 – Keep Pace with AI Developments: Intermediate Level Course 30 takes center stage, offering a deep dive into the rapidly evolving world of artificial intelligence. This course isn’t just about understanding the latest advancements; it’s about equipping you with the skills to navigate this dynamic landscape and contribute meaningfully to the field.
Imagine mastering machine learning algorithms, delving into deep learning techniques, and exploring the potential of natural language processing and computer vision. This course provides the foundation to turn those visions into reality.
The course emphasizes the importance of continuous learning in AI. It equips you with the knowledge and skills needed to understand complex AI applications, analyze industry trends, and contribute to AI projects. Whether you’re looking to transition into a career in AI or simply enhance your understanding of this transformative technology, this intermediate-level course is a valuable asset.
Understanding the Pace of AI Development
The field of artificial intelligence (AI) is evolving at an unprecedented pace, transforming industries and impacting our lives in profound ways. This rapid development is driven by a confluence of factors, including advancements in computing power, the exponential growth of data, and breakthroughs in algorithms.
Key Drivers of AI Development
The rapid evolution of AI can be attributed to several key drivers:
- Increased Computing Power:The development of more powerful and efficient processors, such as GPUs and specialized AI chips, has enabled the training of increasingly complex AI models. These advancements allow AI systems to process vast amounts of data and perform complex computations, leading to significant improvements in accuracy and performance.
- Data Availability:The explosion of data generated by various sources, including social media, sensors, and the internet of things (IoT), has provided AI systems with a rich source of information for training and learning. This abundance of data has been instrumental in driving progress in areas like natural language processing, computer vision, and machine learning.
- Algorithm Advancements:Researchers are continuously developing new and improved algorithms, such as deep learning and reinforcement learning, which enable AI systems to learn from data more effectively and solve increasingly complex problems. These advancements have led to significant breakthroughs in areas like image recognition, speech synthesis, and autonomous driving.
Keeping pace with the rapid advancements in AI can feel like a constant uphill battle. Sometimes, you just need a creative outlet to de-stress. Why not try a fun DIY project like making diy glitter photo frames ?
It’s a great way to unwind and unleash your inner artist, leaving you refreshed and ready to tackle those complex AI algorithms in Course 30.
Recent Breakthroughs in AI
The rapid pace of AI development has resulted in several recent breakthroughs with significant potential implications:
- Generative AI:The emergence of generative AI models, such as DALL-E 2 and Kami, has revolutionized content creation. These models can generate realistic images, text, and even code from simple prompts, opening up new possibilities for creative expression and automation.
- AI in Healthcare:AI is transforming healthcare by enabling faster and more accurate diagnosis, personalized treatment plans, and drug discovery. For example, AI-powered systems can analyze medical images to detect diseases early, predict patient outcomes, and assist in surgical procedures.
- Autonomous Vehicles:Advancements in AI have made autonomous vehicles a reality, with companies like Tesla and Waymo leading the way. These vehicles use AI algorithms to perceive their surroundings, navigate roads, and make driving decisions, promising safer and more efficient transportation.
The Importance of Continuous Learning
The field of AI is constantly evolving, with new breakthroughs and applications emerging regularly. To remain competitive in this dynamic landscape, it is crucial to engage in continuous learning and stay abreast of the latest advancements.
The Challenges of Keeping Pace
The rapid pace of AI development presents significant challenges for individuals and organizations alike. The constant influx of new research papers, algorithms, and tools can be overwhelming. Furthermore, the complexity of AI concepts requires a solid foundation in mathematics, computer science, and related disciplines.
Keeping up with the latest developments necessitates a commitment to lifelong learning.
Strategies for Lifelong Learning in AI
To navigate the challenges of continuous learning in AI, it is essential to adopt effective strategies.
- Attend Conferences and Workshops:AI conferences provide a platform to connect with leading researchers, industry experts, and fellow practitioners. These events offer valuable insights into cutting-edge research, emerging trends, and practical applications of AI.
- Read Research Papers and Publications:Staying current with the latest research is crucial for understanding the direction of AI development. Reading research papers from reputable journals and conferences allows you to gain a deeper understanding of the theoretical underpinnings and practical applications of AI algorithms and techniques.
- Participate in Online Courses and MOOCs:Online learning platforms offer a wide range of courses and tutorials on various aspects of AI, from introductory concepts to advanced techniques. These platforms provide flexibility and accessibility, allowing you to learn at your own pace and convenience.
- Engage in Online Communities and Forums:Online communities and forums dedicated to AI provide a valuable space for discussions, knowledge sharing, and collaboration. Engaging with other professionals in the field allows you to learn from their experiences, exchange ideas, and stay informed about emerging trends.
- Experiment with AI Tools and Frameworks:Hands-on experience is crucial for developing a practical understanding of AI concepts. Experimenting with AI tools and frameworks allows you to apply theoretical knowledge to real-world problems and gain valuable insights into the capabilities and limitations of different AI techniques.
Benefits of an Intermediate-Level AI Course: Keep Pace With Developments Ai With Intermediate Level Course 30
An intermediate-level AI course provides a comprehensive foundation in AI concepts and skills, equipping individuals with the necessary knowledge and abilities to contribute meaningfully to AI projects and understand the complexities of AI applications. It goes beyond introductory concepts, delving deeper into advanced techniques and practical applications.
Key Concepts and Skills Covered
An intermediate-level AI course covers a wide range of key concepts and skills, encompassing both theoretical and practical aspects of AI. These include:
- Machine Learning Algorithms:Understanding the principles and working mechanisms of various machine learning algorithms, such as linear regression, logistic regression, decision trees, support vector machines, and neural networks. This includes learning how to select the appropriate algorithm for different tasks and optimize its performance.
Keeping up with the rapid pace of AI development can feel overwhelming, but an intermediate-level course like “AI 30” can help you gain a solid understanding of the field. After a long day of learning, you deserve some cozy relaxation, and what better way to unwind than by designing your own holiday pajamas?
Create your own holiday pajamas and embrace the festive spirit while you recharge for the next AI learning session.
- Deep Learning Techniques:Exploring the architecture and workings of deep neural networks, including convolutional neural networks (CNNs) for image recognition, recurrent neural networks (RNNs) for sequential data processing, and generative adversarial networks (GANs) for generating realistic data. This involves understanding concepts like backpropagation, gradient descent, and activation functions.
- Natural Language Processing (NLP):Delving into the techniques used for processing and understanding human language, including text classification, sentiment analysis, machine translation, and chatbot development. This involves learning about techniques like tokenization, stemming, lemmatization, and word embedding.
- Computer Vision:Exploring the principles and applications of computer vision, including image classification, object detection, and image segmentation. This involves understanding concepts like feature extraction, image filtering, and deep learning architectures specifically designed for visual data.
- Reinforcement Learning:Understanding the principles of reinforcement learning, where agents learn through trial and error by interacting with their environment. This involves learning about concepts like Markov decision processes, Q-learning, and deep reinforcement learning.
- AI Ethics and Responsible AI:Discussing the ethical implications of AI development and deployment, including bias, fairness, privacy, and transparency. This involves understanding the importance of responsible AI practices and the need for ethical considerations in AI design and implementation.
- AI Tools and Frameworks:Gaining practical experience with popular AI tools and frameworks, such as TensorFlow, PyTorch, scikit-learn, and Keras. This involves learning how to build and deploy AI models using these tools, as well as understanding their capabilities and limitations.
Equipping Individuals for AI Projects
The skills acquired in an intermediate-level AI course empower individuals to make meaningful contributions to AI projects. These skills enable them to:
- Design and Develop AI Solutions:Understand the process of designing and developing AI solutions, from problem definition and data collection to model training and evaluation. This includes being able to select appropriate algorithms, optimize model performance, and deploy models for real-world applications.
- Collaborate with AI Teams:Effectively communicate with other AI professionals, including data scientists, machine learning engineers, and software developers. This involves understanding the roles and responsibilities within an AI team and being able to contribute to collaborative projects.
- Analyze and Interpret AI Results:Critically evaluate the results of AI models, identify potential biases and limitations, and communicate insights effectively to stakeholders. This includes understanding the importance of model interpretability and the need for robust evaluation metrics.
Practical Applications of AI Concepts
The concepts learned in an intermediate-level AI course have numerous practical applications across various industries:
- Healthcare:AI is used for disease diagnosis, drug discovery, personalized medicine, and patient monitoring. Intermediate-level AI skills enable professionals to develop AI-powered tools that can improve healthcare outcomes and efficiency.
- Finance:AI is used for fraud detection, risk assessment, algorithmic trading, and customer service. Intermediate-level AI skills enable professionals to build AI systems that enhance financial operations and decision-making.
- Retail:AI is used for personalized recommendations, inventory management, customer segmentation, and fraud prevention. Intermediate-level AI skills enable professionals to develop AI-powered solutions that optimize retail operations and customer experiences.
- Manufacturing:AI is used for predictive maintenance, quality control, process optimization, and robotics. Intermediate-level AI skills enable professionals to develop AI systems that improve manufacturing efficiency and productivity.
- Transportation:AI is used for autonomous vehicles, traffic optimization, route planning, and logistics. Intermediate-level AI skills enable professionals to contribute to the development of intelligent transportation systems.
Course Structure and Content
An intermediate-level AI course is designed to provide a comprehensive understanding of advanced AI concepts and techniques. It typically involves a structured curriculum, practical assignments, and hands-on projects to solidify learning.
Course Structure
The course structure is designed to progressively build upon foundational knowledge and introduce more complex topics. It typically includes:
- Modules:The course is divided into modules, each focusing on a specific area of AI. Each module covers a range of topics, builds upon previous knowledge, and introduces new concepts and techniques.
- Assignments:To assess understanding and encourage active learning, each module includes assignments. These assignments can range from theoretical exercises to practical coding challenges, allowing students to apply their knowledge to real-world scenarios.
- Projects:The course culminates in one or more comprehensive projects. These projects allow students to integrate their knowledge and skills to develop and implement AI solutions for specific problems.
Course Content
The course content delves into advanced AI concepts and techniques, including:
- Machine Learning Algorithms:This module focuses on various machine learning algorithms, including:
- Supervised Learning:Linear Regression, Logistic Regression, Support Vector Machines, Decision Trees, Random Forests, Naive Bayes, K-Nearest Neighbors.
- Unsupervised Learning:K-Means Clustering, Principal Component Analysis (PCA), Hierarchical Clustering.
- Reinforcement Learning:Q-Learning, Deep Q-Learning, SARSA.
- Deep Learning Techniques:This module explores the world of deep learning, covering:
- Neural Networks:Multilayer Perceptrons (MLPs), Convolutional Neural Networks (CNNs), Recurrent Neural Networks (RNNs), Long Short-Term Memory (LSTM) Networks, Generative Adversarial Networks (GANs).
- Deep Learning Frameworks:TensorFlow, PyTorch, Keras.
- Natural Language Processing (NLP):This module delves into the processing and understanding of human language, including:
- Text Preprocessing:Tokenization, Stemming, Lemmatization, Stop Word Removal.
- Language Models:Recurrent Neural Networks (RNNs), Long Short-Term Memory (LSTM) Networks, Transformers (BERT, GPT-3).
- NLP Tasks:Sentiment Analysis, Text Classification, Machine Translation, Question Answering, Summarization.
- Computer Vision:This module explores the use of AI for analyzing and understanding images and videos, including:
- Image Classification:Identifying objects and scenes in images.
- Object Detection:Locating and identifying objects within images.
- Image Segmentation:Dividing an image into meaningful regions.
- Video Analysis:Understanding and interpreting video content.
Key Concepts and Applications
The following table showcases key concepts covered in each module and their real-world applications:
Module | Key Concepts | Real-World Applications |
---|---|---|
Machine Learning Algorithms | Linear Regression, Logistic Regression, Support Vector Machines, Decision Trees, Random Forests, Naive Bayes, K-Means Clustering, Principal Component Analysis (PCA) | Predictive modeling (e.g., predicting customer churn, stock prices), fraud detection, medical diagnosis, image recognition |
Deep Learning Techniques | Convolutional Neural Networks (CNNs), Recurrent Neural Networks (RNNs), Long Short-Term Memory (LSTM) Networks, Generative Adversarial Networks (GANs) | Image and video analysis (e.g., self-driving cars, facial recognition), natural language processing (e.g., machine translation, chatbot development), speech recognition |
Natural Language Processing (NLP) | Text Preprocessing, Language Models, Sentiment Analysis, Text Classification, Machine Translation | Chatbots, virtual assistants, language translation, sentiment analysis, text summarization |
Computer Vision | Image Classification, Object Detection, Image Segmentation, Video Analysis | Self-driving cars, medical imaging, security systems, retail analytics, robotics |
Practical Applications of AI Concepts
This section delves into the real-world applications of the AI concepts explored in this course. By understanding how these concepts are applied across various industries, you’ll gain a deeper appreciation for the transformative potential of AI and its ability to address complex challenges.
Keeping pace with developments in AI can feel like a constant uphill battle, but an intermediate-level course like “30” can give you the tools you need to navigate this exciting field. It’s important to remember that AI is constantly evolving, just like the way we understand and consume food.
The food strategy initiative highlighting the goodness of Irish foods reminds us of the value in understanding our local resources and adapting to changing trends. Similarly, staying current with AI advancements requires a dedication to learning and adapting, and a course like “30” can be the perfect springboard for that journey.
Applications in Healthcare
The healthcare industry is undergoing a significant transformation driven by AI. AI-powered tools are being used to diagnose diseases, personalize treatment plans, and improve patient outcomes. Here are some specific examples:
- Medical Imaging Analysis:AI algorithms can analyze medical images like X-rays, CT scans, and MRIs to detect abnormalities and assist radiologists in making accurate diagnoses. These algorithms can identify patterns and subtle changes that may be missed by the human eye, leading to earlier detection and more effective treatment.
- Drug Discovery and Development:AI is accelerating the drug discovery process by analyzing vast datasets of chemical compounds and biological information to identify potential drug candidates. This process can significantly reduce the time and cost associated with traditional drug development.
- Personalized Medicine:AI algorithms can analyze patient data, including medical history, genetic information, and lifestyle factors, to create personalized treatment plans. This approach can optimize treatment outcomes and reduce adverse effects.
Applications in Finance
The financial sector is leveraging AI to enhance efficiency, improve risk management, and personalize financial services. Some key applications include:
- Fraud Detection:AI algorithms can analyze transaction data and identify suspicious patterns to detect fraudulent activities in real-time. This helps financial institutions prevent financial losses and protect their customers.
- Credit Risk Assessment:AI models can assess the creditworthiness of borrowers by analyzing various factors, including credit history, income, and spending patterns. This helps lenders make more informed lending decisions and reduce the risk of default.
- Algorithmic Trading:AI algorithms can analyze market data and execute trades automatically, based on predefined strategies. This can improve trading efficiency and potentially generate higher returns.
Applications in Marketing
AI is revolutionizing the way businesses interact with their customers. AI-powered tools are being used to personalize marketing campaigns, optimize advertising spend, and improve customer service.
- Personalized Marketing:AI algorithms can analyze customer data to create personalized marketing messages and offers. This can increase engagement and conversion rates.
- Predictive Analytics:AI models can predict customer behavior and identify potential opportunities for cross-selling and upselling. This helps businesses optimize their marketing strategies and maximize revenue.
- Chatbots:AI-powered chatbots can provide instant customer support and answer common questions. This can improve customer satisfaction and reduce the workload on human customer service agents.
Case Study: AI-Powered Customer Service in Retail
Imagine a large retail company struggling to provide consistent and efficient customer service across multiple channels. They are facing challenges with long wait times, inconsistent responses, and difficulty in resolving complex issues.To address these challenges, the company implements an AI-powered customer service solution.
This solution leverages natural language processing (NLP) and machine learning (ML) to understand customer inquiries, provide accurate answers, and resolve issues quickly and efficiently.The AI system analyzes customer interactions across various channels, including phone calls, emails, and live chat. It learns from past interactions to improve its ability to understand and respond to customer requests.
The system also integrates with the company’s CRM system to provide a comprehensive view of customer data and preferences.This AI-powered solution results in significant improvements in customer service. Wait times are reduced, customer satisfaction increases, and the company is able to resolve complex issues more effectively.
The AI system also helps the company identify areas for improvement and optimize its customer service processes.
Career Opportunities in AI
The field of Artificial Intelligence (AI) is rapidly evolving, creating a surge in demand for skilled professionals. This demand is not limited to individuals with advanced degrees but also extends to those with intermediate-level AI skills. This course equips you with the foundational knowledge and practical skills needed to navigate the exciting world of AI and secure a fulfilling career.
Data Scientist
Data scientists are in high demand across various industries. They leverage their knowledge of statistics, machine learning, and data analysis to extract insights from vast datasets, informing business decisions and driving innovation. The role involves:* Collecting and cleaning data:Data scientists work with raw data, cleaning and preparing it for analysis.
Building and deploying machine learning models
They design and implement machine learning algorithms to predict future outcomes, identify patterns, and automate tasks.
Communicating insights
Data scientists present their findings to stakeholders, using data visualization and clear communication to translate complex information into actionable insights.
Machine Learning Engineer
Machine learning engineers are responsible for building and deploying machine learning models into production environments. They bridge the gap between theoretical AI concepts and practical applications. The role involves:* Developing and training machine learning models:Machine learning engineers select and optimize appropriate algorithms, train them on large datasets, and fine-tune model performance.
Implementing and deploying models
They integrate machine learning models into software applications, ensuring seamless integration and efficient operation.
Monitoring and maintaining models
Machine learning engineers continuously monitor model performance, identify potential issues, and implement necessary updates to ensure optimal performance.
AI Researcher
AI researchers push the boundaries of AI technology by exploring new algorithms, developing novel approaches, and advancing the state-of-the-art. This role requires a strong foundation in mathematics, computer science, and a deep understanding of AI concepts. Key responsibilities include:* Conducting research:AI researchers design and execute experiments, analyze data, and publish their findings in academic journals and conferences.
Developing new AI algorithms
They explore innovative solutions to complex problems, pushing the limits of AI capabilities.
Collaborating with industry partners
AI researchers often collaborate with industry professionals to translate their research into practical applications.
Future Trends in AI
The field of AI is constantly evolving, with new technologies and applications emerging at an unprecedented pace. Understanding these trends is crucial for anyone seeking to stay ahead in the ever-changing landscape of AI. This section will delve into some of the most impactful trends shaping the future of AI, exploring their potential implications and providing real-world examples of their application.
Generative AI, Keep pace with developments ai with intermediate level course 30
Generative AI refers to a category of AI systems capable of creating new content, such as text, images, audio, and video. These systems learn from vast amounts of data and use this knowledge to generate outputs that resemble the training data.
Generative AI is already making waves in various industries. For instance, in the creative realm, tools like DALL-E 2 and Stable Diffusion are revolutionizing image generation, allowing artists and designers to create stunning visuals with unprecedented ease. Generative AI is also transforming content creation in other domains.
For example, Kami, a large language model, can generate realistic and engaging text, making it a powerful tool for content marketing, writing, and even coding.
Ethical AI
As AI systems become more sophisticated and integrated into our lives, the importance of ethical considerations is paramount. Ethical AI focuses on developing and deploying AI technologies responsibly, ensuring fairness, transparency, and accountability.One key aspect of ethical AI is ensuring that AI systems do not perpetuate existing biases.
This requires careful attention to the data used to train these systems and the design of algorithms to minimize bias.Another critical area is data privacy and security. Ethical AI principles emphasize the need for data protection and responsible data collection practices.
AI for Social Good
AI has the potential to address some of the world’s most pressing challenges, from healthcare to climate change. AI for social good focuses on leveraging AI technologies to solve societal problems and improve the lives of people worldwide.For instance, AI is being used to develop personalized healthcare solutions, predict and prevent natural disasters, and optimize resource allocation for sustainable development.Examples of AI for social good include:
- Healthcare:AI-powered diagnostic tools are helping doctors detect diseases earlier and more accurately, leading to improved patient outcomes.
- Climate Change:AI is being used to monitor environmental changes, optimize energy consumption, and develop innovative solutions for climate mitigation.
- Education:AI-powered tutoring systems are providing personalized learning experiences, making education more accessible and effective.