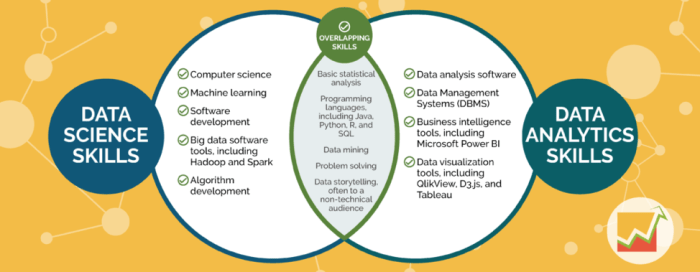
Differences Between BI and AI Analytics
Differences bi ai analytics – Differences Between BI and AI Analytics sets the stage for this enthralling narrative, offering readers a glimpse into a world where data transforms into actionable insights. While both Business Intelligence (BI) and Artificial Intelligence (AI) analytics aim to empower businesses with data-driven decisions, they employ distinct approaches, capabilities, and applications.
This exploration dives deep into the core differences between these two powerful analytical tools, unveiling their strengths, limitations, and future potential.
Imagine a world where businesses can predict future trends, optimize operations, and personalize customer experiences, all powered by the insights gleaned from data. This is the promise of BI and AI analytics, but understanding the differences between these approaches is crucial for harnessing their full potential.
We’ll delve into the core concepts, data handling techniques, predictive capabilities, implementation considerations, and diverse applications of BI and AI analytics, providing a comprehensive understanding of their unique roles in the modern business landscape.
Core Concepts: Differences Bi Ai Analytics
Business Intelligence (BI) and Artificial Intelligence (AI) analytics are both powerful tools for analyzing data and gaining insights. While they share the common goal of extracting valuable information from data, they differ in their approaches, capabilities, and applications.
Differences Between BI and AI Analytics
BI and AI analytics are distinct approaches to data analysis.
- BI analytics focuses on analyzing historical data to understand past trends and patterns. It uses traditional statistical methods and reporting tools to create dashboards and reports that provide insights into business performance.
- AI analytics, on the other hand, leverages machine learning algorithms to identify patterns and make predictions based on both historical and real-time data. It goes beyond simple reporting and can automate complex tasks, such as anomaly detection, forecasting, and customer segmentation.
Objectives and Applications of BI and AI Analytics
The objectives and applications of BI and AI analytics vary based on their underlying principles.
- BI analytics aims to provide actionable insights for decision-making, often focusing on improving operational efficiency, understanding customer behavior, and optimizing resource allocation.
- AI analytics, driven by machine learning, goes beyond insights and seeks to automate tasks, personalize experiences, and make predictions about future events.
Examples of BI and AI Analytics in Industries
BI and AI analytics are widely adopted across various industries.
- Retail: BI analytics is used to analyze sales data, identify customer preferences, and optimize inventory management. AI analytics can predict customer demand, personalize recommendations, and detect fraudulent transactions.
- Healthcare: BI analytics is used to analyze patient data, track disease outbreaks, and optimize resource allocation. AI analytics can diagnose diseases, predict patient outcomes, and personalize treatment plans.
- Finance: BI analytics is used to analyze market trends, assess risk, and manage investments. AI analytics can detect fraud, predict market movements, and personalize financial advice.
- Manufacturing: BI analytics is used to track production processes, identify bottlenecks, and improve efficiency. AI analytics can optimize production schedules, predict equipment failures, and improve quality control.
Predictive and Prescriptive Capabilities
Both BI and AI analytics offer valuable capabilities for predicting future trends and recommending actions. However, their approaches and strengths differ significantly.
Predictive Capabilities Comparison
Predictive analytics uses historical data and statistical models to forecast future outcomes. While both BI and AI can perform predictive analysis, their approaches and capabilities vary.
- BI Predictive Analytics:BI tools typically employ statistical methods like regression analysis and time series forecasting. They excel at identifying patterns and trends within structured data, making them suitable for forecasting sales, inventory levels, and customer churn.
- AI Predictive Analytics:AI models, particularly machine learning algorithms, can analyze vast amounts of structured and unstructured data, including text, images, and audio. They can uncover complex relationships and patterns, enabling more accurate predictions for various scenarios, including fraud detection, customer sentiment analysis, and product demand forecasting.
Understanding the differences between BI and AI analytics is crucial for making informed decisions, much like choosing the right recipe for a healthy meal. If you’re looking for a nutritious and delicious option, try these peas pesto quinoa patties , packed with protein and flavor.
Similarly, when deciding between BI and AI analytics, consider your specific needs and goals to achieve the best results.
Prescriptive Capabilities Comparison
Prescriptive analytics goes beyond prediction by recommending actions based on the analysis. It leverages optimization techniques to identify the best course of action for a given situation.
- BI Prescriptive Analytics:BI tools often use optimization algorithms to recommend inventory levels, pricing strategies, and resource allocation based on predefined constraints and business rules. Their prescriptive capabilities are typically limited to structured data and predefined scenarios.
- AI Prescriptive Analytics:AI models, especially deep learning algorithms, can learn from complex, unstructured data and adapt to dynamic environments. They can recommend personalized marketing campaigns, optimize supply chains, and suggest personalized product recommendations based on individual customer behavior and preferences.
Examples of Predictive and Prescriptive Analytics in Action
- Retail Sales Forecasting:A retail company can use BI tools to forecast future sales based on historical data and seasonal trends. AI models can further enhance these predictions by considering external factors like economic indicators, competitor activity, and social media sentiment.
- Fraud Detection:Financial institutions can leverage AI models to detect fraudulent transactions by analyzing transaction patterns, user behavior, and other data sources. These models can identify anomalies and flag suspicious activities in real-time.
- Customer Churn Prediction:Telecommunication companies can use BI tools to predict customer churn based on factors like call volume, service usage, and contract expiration. AI models can further refine these predictions by analyzing customer sentiment, social media interactions, and other unstructured data.
- Personalized Recommendations:E-commerce platforms can utilize AI models to recommend products based on customer browsing history, purchase history, and other factors. These recommendations can be personalized and tailored to individual preferences, increasing customer engagement and sales.
Accuracy and Reliability of BI and AI Models
The accuracy and reliability of predictions made by BI and AI models depend on several factors, including the quality of data, the complexity of the model, and the specific use case.
Model Type | Accuracy | Reliability | Strengths | Weaknesses |
---|---|---|---|---|
BI Models | Moderate to High | High | Handle structured data well, provide clear explanations, easy to implement | Limited to structured data, less adaptable to dynamic environments, require significant data cleaning |
AI Models | High to Very High | Moderate to High | Analyze complex data, adapt to dynamic environments, handle unstructured data well | Black box nature, require large datasets, prone to bias, can be computationally expensive |
Implementation and Integration
Successfully implementing BI and AI analytics solutions requires careful planning and execution. The integration of these tools with existing business systems presents a significant challenge, but it’s crucial for unlocking the full potential of data-driven decision-making.
The differences between BI and AI analytics are vast, with BI focusing on historical data and AI delving into predictive insights. This is particularly relevant when considering the recent research on OpenAI’s Procgen benchmark overfitting , which highlights the potential for AI models to overfit on specific training data, hindering their ability to generalize to new situations.
Understanding these limitations is crucial for ensuring that AI analytics are used effectively and responsibly.
Challenges and Considerations
Implementing BI and AI analytics solutions involves various challenges, including data quality, integration complexities, and resource constraints.
- Data Quality: The accuracy and completeness of data are crucial for reliable insights. Data cleansing and standardization are essential to ensure data integrity and consistency.
- Integration Complexity: Integrating BI and AI tools with existing systems can be complex, requiring expertise in data integration, APIs, and data warehousing.
- Resource Constraints: Implementing and maintaining these solutions requires skilled personnel, including data scientists, analysts, and IT professionals.
Integration Capabilities
BI and AI tools offer varying levels of integration capabilities.
- BI Tools: Traditional BI tools typically integrate with databases and data warehouses through connectors and APIs. These tools often provide pre-built connectors for popular enterprise systems like ERP, CRM, and databases.
- AI Tools: AI tools are often designed to work with structured and unstructured data sources, including databases, APIs, and cloud storage services. They may leverage machine learning algorithms to analyze data from multiple sources and provide insights.
Best Practices for Integration
Integrating BI and AI analytics solutions effectively requires following best practices.
The differences between BI and AI analytics are fascinating, especially when you consider how they can be applied to everyday tasks. For example, AI could help you automatically categorize your photos, while BI could then be used to analyze the distribution of those photos across different folders.
This brings me to a cool DIY project I recently discovered: how to transfer a photo to wood. It’s a great way to personalize your home décor and showcases the power of technology to create beautiful, tangible results.
Just like how AI can enhance image quality before transfer, BI can be used to analyze the effectiveness of your creative endeavors, ensuring you get the most out of your artistic endeavors.
- Define Clear Objectives: Clearly define the goals and objectives of the integration project to ensure alignment with business needs.
- Establish a Data Governance Framework: Implement data governance policies to ensure data quality, security, and compliance.
- Choose the Right Tools: Select BI and AI tools that are compatible with existing systems and meet the specific needs of the organization.
- Pilot and Iterate: Start with a pilot project to test the integration and refine the process before scaling it across the organization.
- Foster Collaboration: Encourage collaboration between IT, business, and data science teams to ensure successful integration and adoption.
Applications and Use Cases
Both BI and AI analytics have revolutionized how businesses make decisions, offering unique advantages across various industries. BI excels in providing insights into historical data, while AI leverages predictive and prescriptive capabilities to anticipate future trends and optimize operations.
Use Cases Where BI Analytics Excel
BI analytics excels in providing insights into historical data, enabling businesses to understand past trends, identify patterns, and make informed decisions. Here are some common use cases:
- Sales Performance Analysis:BI tools can analyze sales data to identify top-performing products, regions, and salespersons. This information can help businesses optimize sales strategies, allocate resources effectively, and improve overall revenue generation.
- Customer Segmentation:By analyzing customer demographics, purchase history, and engagement data, businesses can segment their customer base into distinct groups with unique needs and preferences. This segmentation allows for targeted marketing campaigns, personalized offers, and improved customer satisfaction.
- Financial Reporting and Analysis:BI tools can aggregate financial data from various sources, providing a comprehensive view of a company’s financial performance. This enables businesses to track key metrics, identify areas for improvement, and make informed financial decisions.
- Operational Efficiency Analysis:By analyzing operational data, businesses can identify bottlenecks, optimize workflows, and improve overall efficiency. This can lead to cost savings, reduced lead times, and increased productivity.
Use Cases Where AI Analytics Offer Unique Advantages
AI analytics, with its ability to learn from data and make predictions, offers unique advantages in scenarios where complex patterns, future trends, and real-time decision-making are crucial.
- Predictive Maintenance:AI algorithms can analyze sensor data from machinery to predict potential failures before they occur. This enables businesses to schedule maintenance proactively, minimizing downtime and reducing repair costs.
- Fraud Detection:AI algorithms can identify suspicious patterns in financial transactions, helping businesses prevent fraud and protect their assets. By analyzing data from multiple sources, AI can detect anomalies and flag potentially fraudulent activities.
- Personalized Recommendations:AI-powered recommendation engines can analyze customer data to provide personalized product suggestions, improving customer engagement and increasing sales. This can be seen in online retailers, streaming services, and other platforms where user preferences are crucial.
- Dynamic Pricing:AI algorithms can analyze real-time market data, competitor pricing, and demand fluctuations to dynamically adjust prices, maximizing revenue and optimizing pricing strategies.
Common Applications of BI and AI Analytics Across Industries, Differences bi ai analytics
Industry | BI Analytics Applications | AI Analytics Applications |
---|---|---|
Retail | Sales performance analysis, customer segmentation, inventory management, pricing optimization | Predictive demand forecasting, personalized recommendations, fraud detection, customer churn prediction |
Healthcare | Patient data analysis, disease outbreak tracking, resource allocation, clinical trial management | Drug discovery and development, personalized medicine, disease prediction, medical imaging analysis |
Finance | Financial reporting, risk assessment, portfolio management, customer relationship management | Fraud detection, credit risk assessment, algorithmic trading, investment portfolio optimization |
Manufacturing | Production planning, quality control, supply chain management, machine performance analysis | Predictive maintenance, process optimization, demand forecasting, inventory management |
Marketing | Campaign performance analysis, customer segmentation, market research, brand sentiment analysis | Targeted advertising, personalized content recommendations, customer churn prediction, lead scoring |
Future Trends and Considerations
The convergence of BI and AI analytics is creating exciting new possibilities for businesses to gain deeper insights and make more informed decisions. This section explores the future trends shaping this landscape, including the ethical considerations surrounding AI analytics and its impact on the future of business intelligence.
Convergence of BI and AI
The future of analytics lies in the seamless integration of BI and AI. This convergence will enable businesses to leverage the power of AI to automate tasks, uncover hidden patterns, and generate actionable insights from massive datasets. AI algorithms can analyze data from various sources, including structured and unstructured data, to identify trends, anomalies, and potential opportunities.
This information can then be integrated into BI dashboards and reports, providing a comprehensive view of business performance and enabling more proactive decision-making.
Ethical Considerations in AI Analytics
As AI analytics becomes increasingly prevalent in business, ethical considerations are paramount. These considerations encompass data privacy, bias in algorithms, and the potential for job displacement.
- Data Privacy:AI algorithms rely on vast amounts of data, raising concerns about data privacy and security. Businesses must ensure they comply with data protection regulations and obtain informed consent from individuals whose data is being used. Transparent data governance practices and robust security measures are crucial to protect sensitive information.
- Algorithmic Bias:AI algorithms can perpetuate existing biases present in the training data. This can lead to unfair or discriminatory outcomes, particularly in areas like hiring, lending, and criminal justice. It is essential to address algorithmic bias through diverse training data, rigorous testing, and ongoing monitoring to ensure fairness and equity.
- Job Displacement:The automation capabilities of AI analytics raise concerns about job displacement. While AI can create new opportunities, it’s crucial to invest in reskilling and upskilling programs to prepare workforces for the changing job market. Businesses must prioritize human-centered AI solutions that enhance, rather than replace, human capabilities.
Impact on the Future of Business Intelligence
AI analytics will significantly transform the future of business intelligence, empowering businesses to gain a competitive advantage by:
- Enhanced Predictive Capabilities:AI algorithms can analyze historical data and identify patterns to predict future outcomes with greater accuracy. This enables businesses to forecast demand, anticipate market trends, and make proactive decisions to optimize operations and mitigate risks.
- Personalized Insights:AI can personalize insights by tailoring information to individual users’ needs and preferences. This allows businesses to deliver targeted recommendations, optimize marketing campaigns, and improve customer satisfaction.
- Automated Insights Generation:AI can automate the process of generating insights from data, freeing up BI professionals to focus on higher-level tasks such as strategic analysis and decision-making. This allows for faster turnaround times and more efficient use of resources.
- Real-time Analytics:AI enables real-time data analysis, allowing businesses to monitor performance, identify issues, and take corrective actions immediately. This agility is crucial in today’s dynamic business environment.