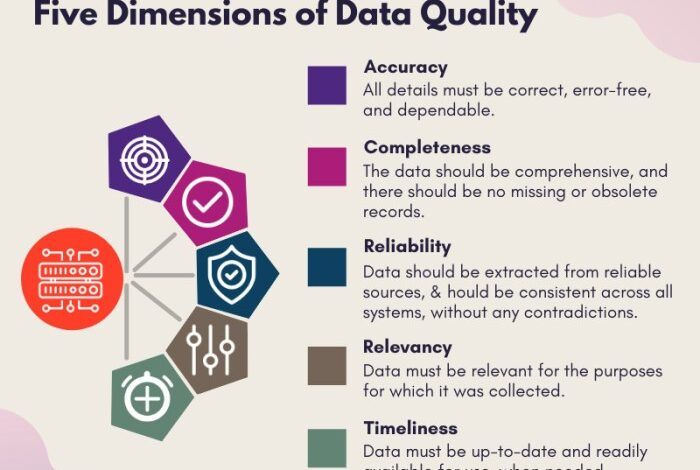
Data Quality vs Data Governance: Building Trust and Control
Data quality vs data governance: These two concepts are often intertwined, but understanding their distinct roles is crucial for any organization aiming to harness the power of data. Data quality, the foundation of trust, ensures that information is accurate, complete, and reliable.
Without it, even the most sophisticated analytics can lead to flawed insights and decisions. Data governance, on the other hand, establishes the rules of the road, defining how data is collected, stored, used, and protected. It’s the framework that ensures accountability and compliance, safeguarding sensitive information and promoting ethical data practices.
Think of it like building a house. Data quality is the foundation – it needs to be solid and stable to support the structure. Data governance is the blueprint, outlining the design, materials, and construction process. Both are essential for creating a secure and functional dwelling.
In the world of data, both data quality and governance are vital for building a strong foundation for decision-making and achieving strategic goals.
Data Quality: The Foundation of Trust
Data quality is the cornerstone of effective decision-making. It refers to the accuracy, completeness, consistency, and timeliness of data, ensuring that it is reliable and trustworthy for analysis and insights. In today’s data-driven world, data quality is paramount, as poor data can lead to inaccurate conclusions, flawed strategies, and ultimately, detrimental business outcomes.
The Impact of Poor Data Quality
Poor data quality can have significant consequences for organizations, leading to a range of problems:
- Inaccurate Insights:Data errors can skew analysis and lead to misleading conclusions, making it difficult to identify trends and patterns. This can result in misguided decisions and missed opportunities.
- Flawed Outcomes:Decisions based on inaccurate data can lead to poor outcomes, such as inefficient operations, ineffective marketing campaigns, and even financial losses.
- Eroded Trust:When stakeholders encounter inaccurate or unreliable data, it can erode trust in the organization’s ability to make informed decisions. This can lead to a loss of credibility and customer confidence.
- Increased Costs:Addressing data quality issues can be time-consuming and costly. It may require significant resources to identify, correct, and prevent errors in the future.
Best Practices for Data Quality
Maintaining high data quality requires a proactive approach and a commitment to best practices:
- Data Accuracy:Ensure that data is free from errors and reflects the true state of affairs. This can involve implementing data validation rules, using data cleansing techniques, and establishing clear data entry guidelines.
- Data Completeness:Ensure that all necessary data fields are filled in and that there are no missing values. This can involve implementing data capture processes that require complete information and using data imputation techniques to fill in missing values.
- Data Consistency:Ensure that data is consistent across different sources and systems. This can involve establishing data standards, using data mapping techniques, and implementing data integration processes.
- Data Timeliness:Ensure that data is up-to-date and reflects the current state of affairs. This can involve implementing data refresh processes, using real-time data feeds, and establishing data retention policies.
Data Validation and Cleansing
Data validation and cleansing are crucial processes for maintaining data quality.
- Data Validation:This process involves checking data against predefined rules and standards to identify errors and inconsistencies. Data validation techniques include range checks, format checks, and uniqueness checks.
- Data Cleansing:This process involves correcting errors and inconsistencies in data. Data cleansing techniques include data standardization, data imputation, and data deduplication.
Data Governance: Data Quality Vs Data Governance
Data governance is the framework that defines how organizations manage, protect, and utilize their data assets. It establishes policies, processes, and controls to ensure data quality, integrity, and compliance with relevant regulations. It is the backbone of data management, providing a structured approach to handling data across the organization.
Key Principles of Data Governance
Data governance revolves around a set of core principles that guide its implementation and ensure its effectiveness. These principles establish a foundation for responsible data management, fostering trust and accountability within the organization.
Data quality and data governance are often seen as separate entities, but they’re more like two sides of the same coin. Data quality ensures accuracy and consistency, while data governance sets the rules for data management. Think of it like the sister style nothing fancy approach to fashion – you need both the right clothes (data quality) and the right way to wear them (data governance) to look your best (make informed decisions).
In the end, both data quality and data governance are essential for making sure your data is reliable and valuable.
- Data Ownership: Clearly defining data ownership responsibilities is crucial. Each data asset should have a designated owner responsible for its quality, security, and compliance. This owner is accountable for managing the data lifecycle, from creation to deletion.
- Access Control: Access to data should be restricted based on the principle of least privilege. Only authorized individuals should have access to specific data sets, and their access should be limited to what they need to perform their duties. This principle helps protect sensitive information and prevents unauthorized access.
- Data Retention Policies: Organizations need to establish data retention policies that specify how long data should be stored and under what conditions. This policy should consider legal and regulatory requirements, as well as business needs. Data retention policies ensure that data is kept for as long as necessary and then disposed of securely.
Data quality and data governance are often intertwined, just like the intricate straps of a Jimmy Choo sandal. Both are essential for building a strong foundation for any business, but it’s important to remember that data governance sets the rules while data quality ensures those rules are followed.
Think of it like reimagining the iconic Jimmy Choo strappy sandals – you can change the design, but the craftsmanship still needs to be impeccable. Just like those sandals, data needs to be both beautiful and functional to truly be valuable.
Importance of Data Governance
Data governance plays a crucial role in protecting sensitive information and ensuring compliance with regulations. It helps organizations mitigate risks associated with data breaches, legal penalties, and reputational damage.
- Data Security: Data governance strengthens data security by implementing access controls, encryption, and other security measures to protect sensitive data from unauthorized access, use, disclosure, disruption, modification, or destruction.
- Data Privacy: Organizations must comply with data privacy regulations, such as the General Data Protection Regulation (GDPR) and the California Consumer Privacy Act (CCPA). Data governance helps organizations establish policies and processes to meet these regulations, ensuring the ethical and responsible handling of personal information.
- Regulatory Compliance: Many industries have specific regulations regarding data management and security. Data governance frameworks ensure compliance with these regulations, reducing the risk of fines and penalties.
Data Governance Framework
A data governance framework provides a structured approach to managing data assets. It Artikels roles, responsibilities, processes, and policies for data management.
- Roles and Responsibilities: The framework should clearly define roles and responsibilities for data management. This includes identifying data stewards, data owners, data analysts, and other stakeholders involved in data governance.
- Data Policies: Data governance frameworks establish data policies that guide data management practices. These policies cover data quality, data security, data privacy, data retention, and other aspects of data management.
- Data Processes: The framework should define processes for data management, including data collection, data storage, data analysis, and data reporting. These processes should be documented and regularly reviewed to ensure effectiveness.
Data Governance Strategy
A data governance strategy addresses data security, privacy, and quality, ensuring the responsible and ethical use of data assets.
Data quality and data governance are often intertwined, but they play distinct roles in ensuring data reliability. Just like carefully choosing the right paint colors for your Christmas lights can make a huge difference in the overall look and feel, data quality focuses on the accuracy, completeness, and consistency of the data itself.
You can check out some creative ideas for painting your Christmas lights here – a bit of a different approach, but it shows how a little effort can make a big impact! Meanwhile, data governance establishes policies and procedures for managing data, ensuring its security, and promoting its responsible use.
- Data Security: The strategy should Artikel measures to protect data from unauthorized access, use, disclosure, disruption, modification, or destruction. This includes implementing access controls, encryption, and other security measures.
- Data Privacy: The strategy should address data privacy regulations, ensuring the ethical and responsible handling of personal information. This includes implementing policies and processes for data collection, use, and disclosure.
- Data Quality: The strategy should define measures to ensure the accuracy, completeness, consistency, and timeliness of data. This includes establishing data quality metrics, implementing data validation processes, and conducting regular data quality audits.
The Interplay of Data Quality and Data Governance
Data quality and data governance, while distinct concepts, are intricately intertwined, forming a symbiotic relationship that ensures the reliability and trustworthiness of data within an organization. Data quality, as we have discussed, provides the foundation for trust, while data governance establishes the framework for managing and controlling data.
Key Areas of Overlap and Reinforcement
The interplay between data quality and data governance is evident in several key areas:
- Data Standards and Policies:Data governance defines data standards and policies, including data quality standards, that guide data collection, storage, and usage. These standards provide a framework for ensuring data accuracy, completeness, consistency, and timeliness, directly impacting data quality.
- Data Ownership and Accountability:Data governance assigns data ownership and accountability, ensuring that individuals are responsible for maintaining data quality within their respective domains. This accountability fosters a culture of data quality awareness and responsibility.
- Data Lifecycle Management:Data governance encompasses the entire data lifecycle, from data acquisition to disposal. This includes data quality checks and remediation processes at each stage, ensuring that data remains accurate and reliable throughout its lifecycle.
- Data Security and Privacy:Data governance mandates security and privacy measures to protect data from unauthorized access, use, or disclosure. These measures contribute to data quality by preventing data corruption or manipulation.
Effective Data Governance Practices Contribute to Improved Data Quality, Data quality vs data governance
Effective data governance practices play a crucial role in enhancing data quality.
- Data Quality Monitoring and Reporting:Data governance frameworks often include mechanisms for monitoring data quality metrics and generating reports. These reports provide insights into data quality issues, enabling organizations to identify and address problems proactively.
- Data Quality Improvement Initiatives:Data governance enables organizations to implement data quality improvement initiatives. These initiatives may involve training data stewards, developing data quality tools, or establishing data quality review processes.
- Data Quality Audits:Data governance mandates periodic data quality audits to assess the effectiveness of data quality management practices. These audits identify areas for improvement and ensure that data quality remains consistent over time.
Data Quality Issues Can Undermine Data Governance Efforts
Conversely, data quality issues can undermine data governance efforts and compromise data integrity.
- Inaccurate Data:Inaccurate data can lead to incorrect decisions, wasted resources, and reputational damage. This undermines the effectiveness of data governance by compromising the reliability of data used for decision-making.
- Incomplete Data:Incomplete data can hinder data analysis and reporting, making it difficult to draw accurate conclusions. This can disrupt data governance processes by limiting the availability of reliable data for decision-making.
- Inconsistent Data:Inconsistent data can create confusion and ambiguity, leading to errors and inconsistencies in data analysis. This can undermine data governance by creating conflicting data sources and making it challenging to manage data effectively.
- Outdated Data:Outdated data can provide an inaccurate picture of current conditions, leading to poor decisions. This can compromise data governance by using unreliable data for decision-making.
Building a Data-Driven Culture
A data-driven culture is not merely about collecting and analyzing data; it’s about using data to inform decisions, drive innovation, and improve performance across all aspects of an organization. It requires a fundamental shift in mindset, where data becomes the cornerstone of strategic thinking and operational efficiency.
Strategies for Fostering a Data-Driven Culture
To cultivate a data-driven culture, organizations need to implement practical strategies that encourage data literacy, empower employees, and promote a data-centric approach to decision-making.
- Leadership Buy-In:Data-driven decision-making starts at the top. Leaders must champion the importance of data, actively use data in their own decision-making, and allocate resources to support data initiatives. They need to be vocal advocates for data-driven approaches and set clear expectations for data utilization across the organization.
- Data Accessibility and Transparency:Make data readily available and accessible to all employees who need it. Implement data visualization tools and dashboards that present insights in a clear and understandable manner. Encourage open communication and collaboration around data, fostering a culture of transparency and trust.
- Data Literacy Programs:Investing in data literacy training programs for employees at all levels is crucial. These programs should cover fundamental data concepts, data analysis techniques, and the interpretation of data visualizations. The goal is to empower employees to understand, analyze, and utilize data effectively in their daily work.
- Data-Driven Performance Metrics:Define key performance indicators (KPIs) that are aligned with organizational goals and track them regularly. Use data to monitor progress, identify areas for improvement, and make data-informed adjustments to strategies and operations.
- Data-Driven Decision-Making Processes:Integrate data analysis into decision-making processes at all levels. Encourage employees to present data-backed recommendations and support their arguments with data insights. Foster a culture where data is used to challenge assumptions, validate hypotheses, and make more informed decisions.
Roles and Responsibilities in Data Quality and Governance
Effective data governance requires a clear understanding of roles and responsibilities for different stakeholders. This ensures accountability, coordination, and efficient management of data quality and governance initiatives.
Role | Responsibilities |
---|---|
Data Owner | Defines data requirements, ensures data accuracy, and oversees data quality and governance within their domain. |
Data Steward | Manages the data lifecycle, including data collection, storage, and use. Ensures data integrity and compliance with data policies. |
Data Analyst | Analyzes data, identifies trends and patterns, and provides insights to support decision-making. |
Data Scientist | Develops predictive models and algorithms, uses advanced statistical techniques to extract valuable insights from data. |
Data Engineer | Designs, builds, and maintains data infrastructure, ensuring data availability and performance. |
IT Security Team | Protects data from unauthorized access, ensuring data security and compliance with regulations. |
Importance of Data Literacy and Training Programs
Data literacy is the ability to understand, interpret, and use data effectively. It’s essential for employees at all levels, from entry-level staff to senior executives. Data literacy programs can empower employees to:
- Make Informed Decisions:Data literacy enables employees to analyze data, identify trends, and make informed decisions based on reliable information. This leads to better decision-making, improved efficiency, and reduced risks.
- Identify and Address Data Quality Issues:Employees with data literacy skills can recognize data quality issues, such as inconsistencies, errors, or missing data. They can proactively address these issues, ensuring the integrity and reliability of the data used for decision-making.
- Collaborate Effectively with Data Professionals:Data literacy allows employees to communicate effectively with data analysts, scientists, and engineers. They can articulate their data needs, understand the insights provided, and contribute to data-driven initiatives.
- Drive Innovation and Continuous Improvement:Data literacy fosters a culture of continuous improvement by enabling employees to analyze data, identify opportunities for optimization, and develop innovative solutions based on data insights.
Data Governance Communication Plan
A comprehensive communication plan is crucial for engaging and informing stakeholders about data governance initiatives. The plan should Artikel key messages, target audiences, and communication channels.
- Define Key Messages:Clearly articulate the purpose, benefits, and key principles of data governance. Emphasize the importance of data quality, data security, and data compliance.
- Identify Target Audiences:Segment stakeholders based on their roles and interests. Tailor communication messages to address the specific needs and concerns of each group.
- Select Communication Channels:Utilize a mix of communication channels, including emails, intranet updates, presentations, workshops, and town hall meetings. Choose channels that are effective for reaching the target audience.
- Establish Communication Cadence:Regularly communicate updates and progress on data governance initiatives. Provide clear timelines, milestones, and key achievements.
- Encourage Feedback and Dialogue:Create opportunities for stakeholders to provide feedback, ask questions, and participate in discussions. This fosters transparency, builds trust, and ensures that data governance initiatives are aligned with stakeholder needs.
Real-World Applications of Data Quality and Data Governance
Data quality and governance are not just theoretical concepts; they are crucial for businesses across various industries. From healthcare to finance, these principles are being implemented to enhance decision-making, improve customer experience, and gain a competitive edge.
Successful Implementation in Various Industries
The successful implementation of data quality and governance varies across different industries. Here are some examples:
- Healthcare: In the healthcare industry, accurate patient data is critical for diagnosis, treatment, and research. Hospitals and clinics are implementing data quality initiatives to ensure the accuracy of patient records, medical history, and billing information. Data governance frameworks are established to comply with regulations like HIPAA and to protect sensitive patient information.
- Finance: Financial institutions rely heavily on data for risk management, fraud detection, and customer profiling. Data quality initiatives focus on ensuring the accuracy and completeness of financial transactions, customer data, and market data. Data governance frameworks are established to comply with regulations like GDPR and to protect customer financial information.
- Retail: Retailers use data to personalize customer experiences, optimize inventory management, and improve marketing campaigns. Data quality initiatives focus on ensuring the accuracy of customer data, product information, and sales data. Data governance frameworks are established to protect customer privacy and to comply with regulations like CCPA.
Challenges and Opportunities in the Age of Big Data and Cloud Computing
The emergence of big data and cloud computing presents both challenges and opportunities for data quality and governance.
- Data Volume and Velocity: The sheer volume and velocity of data generated in the digital age make it challenging to ensure data quality and governance. Traditional data management approaches may not be sufficient to handle the scale and complexity of modern data environments.
- Data Integration and Interoperability: As organizations adopt cloud-based solutions and integrate data from multiple sources, ensuring data consistency and interoperability becomes crucial. Data governance frameworks need to address data integration challenges and establish clear data ownership and accountability.
- Data Security and Privacy: Data security and privacy are paramount in the age of big data. Data governance frameworks must address data protection regulations, implement strong access controls, and ensure data confidentiality, integrity, and availability.
Case Studies Demonstrating Tangible Benefits
Several case studies highlight the tangible benefits of prioritizing data quality and governance:
- A leading retailerimplemented a data quality program to improve the accuracy of customer data. This resulted in a significant reduction in marketing campaign costs and an increase in customer engagement.
- A financial institutionimplemented a data governance framework to comply with regulatory requirements and protect customer financial information. This improved risk management, reduced fraud, and enhanced customer trust.
- A healthcare providerimplemented a data quality initiative to improve the accuracy of patient records. This led to more accurate diagnoses, better treatment outcomes, and reduced medical errors.
Impact on Business Operations, Customer Experience, and Competitive Advantage
Data quality and governance have a significant impact on business operations, customer experience, and competitive advantage:
- Improved Decision-Making: Accurate and reliable data empowers organizations to make informed decisions based on real-time insights.
- Enhanced Customer Experience: Data quality and governance enable organizations to personalize customer experiences, improve customer service, and build stronger customer relationships.
- Increased Efficiency and Productivity: Data quality and governance streamline business processes, reduce errors, and improve operational efficiency.
- Competitive Advantage: Organizations that prioritize data quality and governance gain a competitive edge by leveraging data to innovate, optimize operations, and deliver superior customer experiences.