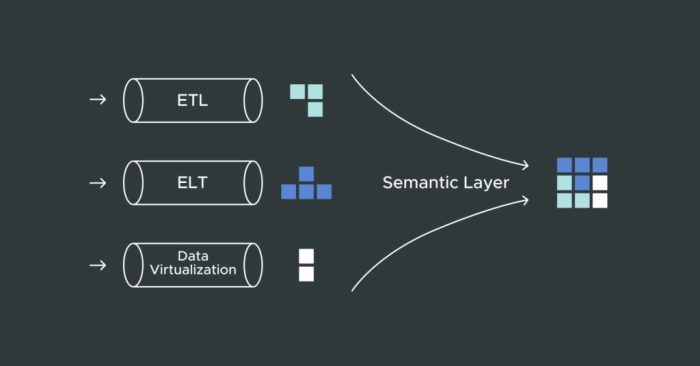
Data Integration vs ETL: Unlocking Datas Potential
Data integration vs ETL: these two terms are often thrown around in the data world, but what do they really mean? Imagine you’re a detective trying to solve a complex case. You need to gather clues from different sources, analyze them, and then put them all together to form a complete picture.
Data integration and ETL are like the tools that help you do just that, allowing you to combine data from various sources and transform it into actionable insights.
Data integration is the process of bringing together data from different sources, whether they’re databases, spreadsheets, APIs, or even social media feeds. It’s about creating a unified view of your data, so you can get a holistic understanding of your business.
ETL, on the other hand, is a specific process that involves extracting data from its source, transforming it into a usable format, and then loading it into a target system. Think of it as the detective’s toolkit – it helps you clean up the messy clues, organize them, and prepare them for analysis.
Data Integration: Data Integration Vs Etl
In today’s data-driven world, organizations are constantly seeking ways to gain valuable insights from their data. However, data is often scattered across multiple sources, making it difficult to analyze and derive meaningful conclusions. This is where data integration comes into play.
Data integration is the process of combining data from various sources into a unified view, creating a comprehensive and consistent data set that can be used for analysis, reporting, and decision-making.
Different Data Sources
Data integration involves bringing together data from various sources, including:
- Operational databases:These systems store data related to daily business operations, such as customer transactions, inventory levels, and sales records.
- Legacy systems:Older systems that may not be easily integrated with newer technologies but still contain valuable data.
- External data sources:Data obtained from third-party providers, such as weather data, market research, or social media analytics.
- Cloud-based applications:Software-as-a-Service (SaaS) applications, such as CRM, marketing automation, and accounting systems, store data in their respective cloud environments.
- Data lakes:Large repositories that store raw data in its native format, providing a central location for data from diverse sources.
Challenges of Data Integration
Integrating data from multiple sources presents several challenges:
- Data quality:Inconsistent data formats, missing values, and duplicate entries can hinder the effectiveness of data integration.
- Data governance:Ensuring data security, privacy, and compliance with regulations is crucial during data integration.
- Data consistency:Different data sources may use different terminologies, units of measurement, and data structures, leading to inconsistencies.
- Scalability:Integrating large volumes of data from diverse sources requires robust infrastructure and efficient processing capabilities.
Benefits of Effective Data Integration, Data integration vs etl
Effective data integration offers numerous benefits:
- Improved data visibility:A unified view of data provides a comprehensive understanding of business operations and customer behavior.
- Enhanced decision-making:Access to integrated data enables data-driven decisions based on accurate and complete information.
- Increased efficiency:Automation of data integration processes reduces manual effort and streamlines data management tasks.
- Improved customer experience:By integrating customer data from different sources, organizations can provide personalized experiences and better customer service.
- Competitive advantage:Organizations that effectively integrate data can gain valuable insights and make better business decisions, leading to a competitive edge.
Data integration and ETL are both essential for making sense of data, but they operate on different levels. Data integration is the broader process of combining data from various sources, while ETL focuses on transforming and loading data into a target system.
Think of it like trying out new Nespresso blends – you might discover a new favorite – ETL helps you prepare the coffee beans (data) for brewing (analysis), while data integration is the process of gathering all the different beans (data sources) together.
Data integration and ETL (Extract, Transform, Load) are crucial for making sense of the vast amounts of data we collect. It’s all about bringing data together from different sources, cleaning it up, and making it ready for analysis. But amidst this data-driven world, news of Sam Altman being ousted from OpenAI sam altman ousted openai reminds us that even in the realm of AI, human decisions and power dynamics play a significant role.
It’s a reminder that the process of data integration and ETL is not just about technical prowess, but also about navigating the complex landscape of leadership, ethics, and the future of technology.
Data integration and ETL (Extract, Transform, Load) are often seen as synonymous, but they’re actually distinct processes. Data integration focuses on bringing together data from various sources, while ETL handles the transformation and loading of data into a target system.
Think of it like the difference between curating a collection of beautiful objects and organizing them into a cohesive display – like the stunning magazine a beautiful mess zooey magazine. Both processes are crucial for making sense of data and leveraging its potential, just as the magazine’s design and content work together to create a compelling visual narrative.