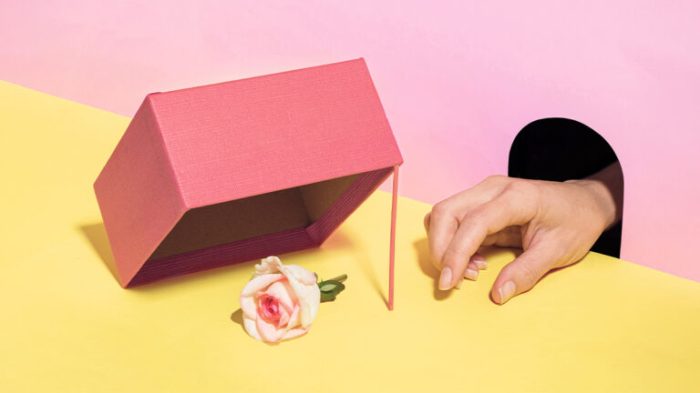
Generative AI Costly Mistakes Tech Buyers Should Avoid
Generative AI Costly Mistakes Tech Buyers Should Avoid sets the stage for this enthralling narrative, offering readers a glimpse into a story that is rich in detail with personal blog style and brimming with originality from the outset.
Generative AI, with its ability to create realistic text, images, and even code, has become a hot topic in the tech world. But while the potential benefits are undeniable, many tech buyers are making costly mistakes in their rush to adopt this revolutionary technology.
From overestimating capabilities to neglecting integration challenges, these pitfalls can derail even the most promising generative AI projects.
Understanding Generative AI
Generative AI is a revolutionary technology that has the potential to transform various industries. It encompasses a range of algorithms and techniques that can create new content, such as text, images, audio, and video, based on existing data. This technology has the ability to learn patterns and relationships from vast amounts of data and generate novel outputs that are similar in style and content to the training data.Generative AI has the potential to unlock significant benefits for businesses, empowering them to automate tasks, enhance creativity, and improve customer experiences.
By leveraging the power of generative AI, organizations can streamline workflows, create engaging content, and gain a competitive edge.
One of the biggest mistakes tech buyers make with generative AI is not understanding the long-term costs involved. It’s easy to get caught up in the hype and forget about the ongoing expenses, like data storage and maintenance. But, just like with any passion, it’s important to nurture the growth.
For gardening enthusiasts, a great resource for finding thoughtful gifts is gifting ideas for gardening enthusiasts farrar and tanner. Similarly, with generative AI, a well-planned investment strategy can lead to a fruitful return, just like a carefully tended garden.
Applications of Generative AI
Generative AI has a wide range of applications across various industries. Here are some notable examples:
- Text Generation:Generative AI models can generate high-quality written content, such as articles, blog posts, social media posts, and even creative fiction. Tools like GPT-3 and LaMDA are capable of producing human-like text that can be used for marketing, content creation, and customer support.
- Image Generation:Generative AI models can create realistic images from scratch or modify existing images. Tools like DALL-E 2 and Stable Diffusion can generate images based on text descriptions, allowing users to visualize their ideas and create unique visual content.
- Audio Generation:Generative AI models can synthesize audio, such as music, speech, and sound effects. Tools like Jukebox and WaveNet can generate music in different genres and styles, while text-to-speech models can convert text into natural-sounding speech.
- Video Generation:Generative AI models can create videos from scratch or manipulate existing videos. Tools like GANs (Generative Adversarial Networks) can generate realistic videos based on input data, enabling applications in film production, advertising, and education.
Costly Mistakes in Generative AI Adoption
Generative AI, with its ability to create novel content, has captured the attention of businesses across industries. However, the rush to adopt these technologies without careful consideration can lead to costly mistakes. This section explores common pitfalls in evaluating generative AI solutions, the risks of overestimating their capabilities, and the potential challenges in integrating them into existing workflows.
Pitfalls in Evaluating Generative AI Solutions
Evaluating generative AI solutions requires a nuanced approach, going beyond flashy demos and marketing hype. Here are some common pitfalls to avoid:
- Focusing solely on output quality:While output quality is crucial, it’s only one aspect of a successful generative AI implementation. Evaluating the model’s training data, bias, and ethical implications is equally important. For example, a generative AI model trained on biased data could produce outputs that perpetuate harmful stereotypes.
- Ignoring the cost of data:Generative AI models require vast amounts of training data, which can be expensive to acquire, clean, and label. Businesses need to factor in these costs when evaluating the feasibility of implementing generative AI.
- Underestimating the need for human oversight:Generative AI models are not perfect and can produce inaccurate or misleading outputs. Human oversight is crucial to ensure the quality and reliability of the generated content. Businesses need to have a clear understanding of how they will integrate human review into their workflows.
- Overlooking the potential for security risks:Generative AI models can be vulnerable to adversarial attacks, where malicious actors manipulate the model’s outputs to generate harmful content. Businesses need to consider security measures to mitigate these risks.
Overestimating the Capabilities of Generative AI, Generative ai costly mistakes tech buyers
Generative AI is a powerful tool, but it’s important to recognize its limitations. Overestimating its capabilities can lead to unrealistic expectations and ultimately disappointment.
- Expecting AI to replace human creativity:Generative AI can assist with creative tasks, but it cannot replace the human element of creativity. It can generate ideas, but it lacks the critical thinking and emotional intelligence to truly understand and express human creativity.
- Assuming AI will always produce perfect outputs:Generative AI models are trained on data, and their outputs are only as good as the data they are trained on. They can make mistakes, generate biased content, or produce outputs that are not aligned with the user’s intent. Businesses need to have realistic expectations and be prepared for occasional errors.
- Ignoring the need for ongoing maintenance and updates:Generative AI models require ongoing maintenance and updates to ensure their performance and accuracy. This includes retraining the model on new data, addressing biases, and improving its overall performance. Businesses need to factor in the costs of ongoing maintenance when evaluating the feasibility of implementing generative AI.
Challenges in Integrating Generative AI into Existing Workflows
Integrating generative AI into existing workflows can be challenging, requiring careful planning and execution.
Generative AI is a powerful tool, but like any powerful tool, it can be misused. Tech buyers need to be careful not to fall into the trap of thinking that AI can solve all their problems. For example, it’s easy to get caught up in the hype around AI-powered chatbots, but it’s important to remember that they’re still just tools.
A good analogy is how a headband can be a stylish accessory, like the iconic brigitte bardot headband hair look, but it can’t fix a bad haircut. Similarly, AI can’t fix underlying business problems, it can only help you address them more efficiently.
- Ensuring compatibility with existing systems:Generative AI models need to be integrated with existing systems and workflows. This can involve modifying existing systems, developing new interfaces, or adopting new data formats. Businesses need to carefully assess the compatibility of generative AI solutions with their existing infrastructure.
- Training employees on new tools and processes:Integrating generative AI into workflows requires training employees on new tools and processes. This can involve providing training materials, offering hands-on workshops, or hiring specialized staff. Businesses need to invest in employee training to ensure successful adoption of generative AI.
Generative AI is a powerful tool, but it’s easy to make costly mistakes when buying into the hype. Just like how you wouldn’t blindly purchase a rare antique without doing your research, you need to carefully consider the specific needs and capabilities of generative AI before investing.
For example, the Forzieri Taoist treasures in Taipei are a testament to the value of historical artifacts, but without understanding their context and significance, they’re just beautiful objects. Similarly, generative AI can be a powerful tool, but without understanding its limitations and potential pitfalls, it can lead to costly mistakes.
- Addressing potential ethical concerns:Generative AI raises ethical concerns related to bias, privacy, and intellectual property. Businesses need to develop policies and procedures to address these concerns and ensure responsible use of generative AI.
Avoiding Costly Mistakes: Generative Ai Costly Mistakes Tech Buyers
Generative AI, with its transformative potential, is rapidly gaining traction across industries. While the benefits are undeniable, navigating the complexities of implementation requires a strategic approach. This section delves into practical steps to ensure a successful and cost-effective integration of generative AI into your organization.
Evaluating Generative AI Suitability
Before embarking on a generative AI journey, a thorough evaluation of its suitability for specific needs is crucial. This involves understanding the potential benefits, limitations, and potential risks associated with generative AI.
- Identify Specific Use Cases:Clearly define the tasks or problems you aim to solve with generative AI. For instance, automating content creation, improving customer service responses, or accelerating drug discovery.
- Assess Data Availability and Quality:Generative AI models thrive on large datasets. Evaluate if you have access to sufficient, relevant, and high-quality data to train and validate the models.
- Consider Resource Requirements:Generative AI models can be computationally intensive. Assess your infrastructure capabilities and potential costs associated with hardware, software, and specialized skills.
- Evaluate Ethical Considerations:Address potential ethical concerns, such as bias in generated content, intellectual property rights, and data privacy.
Conducting Due Diligence
Once you’ve identified suitable use cases, a comprehensive due diligence process helps select the right generative AI solutions. This involves evaluating various vendors and their offerings.
- Vendor Evaluation:Research potential vendors, assessing their experience, expertise, and track record in generative AI. Consider factors like security, compliance, and customer support.
- Solution Assessment:Evaluate the specific features and capabilities of each generative AI solution. Consider factors like model accuracy, customization options, and integration with existing systems.
- Cost Analysis:Compare pricing models, including licensing fees, training costs, and ongoing maintenance expenses.
- Proof of Concept (POC):Conduct a pilot project to test the chosen solution in a real-world setting. This helps validate the feasibility and effectiveness of the solution before full-scale deployment.
Designing a Roadmap for Implementation
A well-defined roadmap ensures a smooth and successful implementation of generative AI. This roadmap should Artikel key milestones, potential risks, and mitigation strategies.
- Establish Clear Goals and Objectives:Define measurable goals and objectives for the generative AI project. For example, improving customer satisfaction, reducing operational costs, or increasing productivity.
- Identify Key Stakeholders:Involve relevant stakeholders from different departments to ensure buy-in and support throughout the implementation process.
- Develop a Training Strategy:Train employees on how to effectively use and manage generative AI tools and technologies.
- Plan for Monitoring and Evaluation:Establish metrics to track the performance of the generative AI solution and make adjustments as needed.
The Impact of Generative AI on Business Operations
Generative AI, with its ability to create novel content, is poised to revolutionize how businesses operate across various functions. From automating tasks to enhancing customer experiences, generative AI offers a wide range of possibilities for businesses to innovate and improve efficiency.
Impact on Various Business Functions
Generative AI can significantly impact various business functions, including:
- Marketing and Sales: Generate personalized marketing campaigns, product descriptions, and social media content. Develop chatbots for customer service and lead generation.
- Content Creation: Automate content creation for websites, blogs, articles, and social media posts. Generate scripts for videos and presentations.
- Research and Development: Analyze large datasets to identify trends and patterns. Generate hypotheses and design experiments.
- Customer Service: Provide personalized customer support through AI-powered chatbots and virtual assistants.
- Human Resources: Automate tasks like resume screening and job description generation. Create personalized training materials for employees.
- Finance: Analyze financial data to identify patterns and predict future trends. Automate tasks like report generation and fraud detection.
- Operations: Optimize supply chains and logistics. Improve efficiency in manufacturing and production processes.
Benefits and Challenges of Using Generative AI
The adoption of generative AI presents both benefits and challenges for businesses.
Benefits
- Increased Efficiency: Automating tasks through generative AI can significantly improve efficiency and reduce the time required for completing certain processes.
- Enhanced Creativity: Generative AI can assist in generating creative ideas and solutions, leading to new products, services, and marketing campaigns.
- Improved Customer Experience: AI-powered chatbots and virtual assistants can provide personalized customer service and support, enhancing the overall customer experience.
- Data-Driven Insights: Generative AI can analyze large datasets to identify patterns and trends, providing valuable insights for decision-making.
Challenges
- Bias and Fairness: Generative AI models can inherit biases from the data they are trained on, leading to unfair or discriminatory outcomes.
- Data Privacy and Security: Generative AI requires access to large datasets, raising concerns about data privacy and security.
- Job Displacement: Automation of tasks through generative AI could lead to job displacement in certain sectors.
- Lack of Transparency: The decision-making processes of generative AI models can be complex and difficult to understand, leading to a lack of transparency.
Ethical Considerations
The deployment of generative AI raises several ethical considerations, including:
- Bias and Discrimination: It is crucial to ensure that generative AI models are not perpetuating existing biases and discriminations.
- Data Privacy and Security: Protecting user data and ensuring responsible use of data in generative AI models is essential.
- Job Displacement: The potential for job displacement through automation requires careful consideration and strategies to mitigate its impact.
- Transparency and Explainability: The decision-making processes of generative AI models should be transparent and explainable to ensure accountability and trust.
Case Studies
Generative AI is rapidly changing the way businesses operate. While the potential benefits are significant, it’s crucial to learn from both the successes and failures of early adopters. Examining real-world case studies helps us understand the practical implications of implementing generative AI and avoid common pitfalls.
Successful Generative AI Implementations
Here are some notable examples of successful generative AI implementations:
- Netflix:Netflix utilizes generative AI for content recommendation. By analyzing user data and viewing history, the platform generates personalized recommendations, significantly improving user engagement and satisfaction. Netflix’s success in this area demonstrates the power of generative AI to enhance user experience and drive revenue.
- Duolingo:Duolingo uses generative AI to personalize language learning experiences. By analyzing user progress and providing adaptive feedback, the platform creates a more engaging and effective learning environment. This case study highlights the potential of generative AI to optimize education and learning processes.
- Jasper.ai:Jasper.ai is a popular generative AI platform for content creation. It enables users to generate high-quality marketing copy, website content, and social media posts. The platform’s success showcases the potential of generative AI to automate content creation and improve efficiency.
Failed Generative AI Projects
While many generative AI projects have been successful, some have encountered challenges. Here are some common reasons for failure:
- Lack of Clear Objectives:Without a clear understanding of the desired outcomes, it’s difficult to assess the success of a generative AI project. It’s crucial to define specific goals and metrics to measure progress.
- Insufficient Data:Generative AI models rely on vast amounts of data for training. Insufficient or biased data can lead to inaccurate results and limit the model’s effectiveness. It’s important to ensure the data used for training is relevant, diverse, and of high quality.
- Integration Challenges:Integrating generative AI into existing systems can be complex and time-consuming. Without careful planning and execution, integration can lead to delays and hinder project success.
- Lack of Expertise:Implementing generative AI requires specialized knowledge and skills. Without a team with the necessary expertise, it’s challenging to develop and deploy effective solutions.
Lessons Learned from Generative AI Successes and Failures
Analyzing successful and failed generative AI projects reveals valuable lessons for future implementations:
- Clear Objectives and Metrics:Establish specific goals and measurable metrics to track progress and evaluate success.
- High-Quality Data:Ensure the data used for training is relevant, diverse, and of high quality.
- Strategic Integration:Plan and execute the integration of generative AI into existing systems carefully to avoid disruptions and delays.
- Skilled Team:Assemble a team with the necessary expertise in generative AI, data science, and software development.
- Ethical Considerations:Address potential ethical concerns associated with generative AI, such as bias, privacy, and the responsible use of technology.