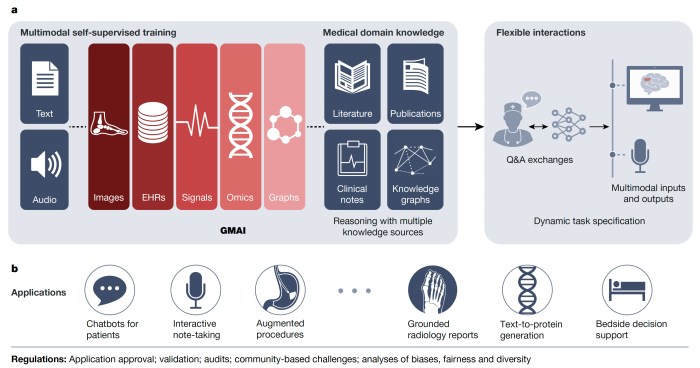
Public or Proprietary Generative AI: Which One Is Right for You?
Public or proprietary generative AI – the choice between these two paths can be a game-changer for businesses and individuals alike. These powerful tools, capable of generating text, images, and even code, offer incredible potential for innovation and efficiency.
But with great power comes great responsibility, and understanding the differences between these two approaches is crucial to making informed decisions.
Public generative AI models, often open-source and freely accessible, allow for collaboration and rapid development. However, they might come with security concerns and limited customization. Proprietary models, on the other hand, offer tailored solutions and enhanced security, but at the cost of flexibility and potentially higher costs.
This blog post delves into the pros and cons of each approach, exploring their applications, challenges, and future trends.
Understanding Public vs. Proprietary Generative AI
Generative AI models are revolutionizing various industries, from content creation to scientific research. These models can generate text, images, audio, and even code, pushing the boundaries of what’s possible with artificial intelligence. However, understanding the differences between public and proprietary generative AI models is crucial for making informed decisions about their use.
Licensing and Access Restrictions
Licensing and access restrictions significantly impact how users can interact with public and proprietary AI models.
- Public generative AI models are typically open-source, meaning their code and training data are freely available for anyone to use, modify, and distribute. This open access fosters collaboration and innovation, allowing developers to build upon existing models and contribute to their improvement.
- Proprietary generative AI models, on the other hand, are owned and controlled by specific companies or organizations. These models are typically subject to licensing agreements, which may restrict access, usage, and distribution. Companies often prioritize monetization, charging users for access or usage, or offering specific services based on the model’s capabilities.
Examples of Public and Proprietary AI Models
Several examples of public and proprietary generative AI models illustrate the differences between these two categories.
- Public:
- GPT-3 (Generative Pre-trained Transformer 3):Developed by OpenAI, GPT-3 is a powerful language model capable of generating human-quality text, translating languages, writing different kinds of creative content, and answering your questions in an informative way. It is available through an API and has been used for various applications, from chatbots to content generation.
- Stable Diffusion:An open-source text-to-image AI model developed by Stability AI, Stable Diffusion allows users to generate images from text prompts. Its open-source nature has fostered a vibrant community of developers and artists, leading to various creative applications and modifications of the model.
The debate about public vs. proprietary generative AI reminds me of the amazing transformation Todd made to his home studio, which you can check out here. Just like Todd’s studio went from cluttered to sleek, the potential of generative AI is vast, and we’re still figuring out the best way to unlock it – openly or behind closed doors.
- Proprietary:
- DALL-E 2:Developed by OpenAI, DALL-E 2 is a text-to-image AI model that generates realistic images from natural language descriptions. It is a powerful tool for creative professionals and designers, allowing them to visualize ideas and create unique visuals.
- Midjourney:A proprietary AI art generator that uses a neural network to create images from text descriptions. It is known for its high-quality and artistic outputs, attracting a large community of artists and designers. Midjourney is accessible through a paid subscription service.
Benefits of Public Generative AI
Publicly available generative AI models offer numerous advantages, empowering researchers, developers, and businesses alike to harness the power of AI in innovative ways. These models provide a valuable resource for pushing the boundaries of AI research and fostering collaboration within the AI community.
Open-Source Development and Community Collaboration
Open-source development plays a crucial role in the advancement of public generative AI models. The collaborative nature of open-source projects allows researchers and developers worldwide to contribute to the development and improvement of these models. This fosters a vibrant ecosystem where knowledge is shared, and ideas are freely exchanged.
The debate between public and proprietary generative AI models is fascinating. On one hand, public models offer accessibility and collaboration, allowing anyone to explore their capabilities. On the other hand, proprietary models often boast advanced features and greater control. This reminds me of the nesting simple song lyrics painting project, where artists utilize readily available resources to create unique and captivating works.
Ultimately, the choice between public and proprietary generative AI depends on individual needs and priorities.
- Rapid Innovation:Open-source models benefit from the collective efforts of numerous contributors, leading to rapid advancements in AI capabilities. New algorithms, architectures, and training techniques are constantly being developed and integrated into these models, pushing the boundaries of what AI can achieve.
- Transparency and Trust:The open-source nature of these models promotes transparency and trust. Users can examine the model’s code, understand its workings, and identify potential biases or limitations. This transparency is essential for building confidence in AI systems and ensuring their responsible use.
- Accessibility and Democratization:Public generative AI models make AI technology accessible to a wider audience, including researchers, developers, and even individuals without extensive technical expertise. This democratization of AI empowers more people to explore and utilize AI for various purposes, driving innovation across diverse fields.
Examples of Public AI Models for Research and Innovation
Public generative AI models have been instrumental in driving research and innovation in various domains. These models have enabled breakthroughs in areas such as natural language processing, computer vision, and drug discovery.
- Natural Language Processing:Models like GPT-3 and BERT have revolutionized natural language processing tasks, enabling more sophisticated language understanding and generation capabilities. These models have been used to develop chatbots, language translation tools, and text summarization systems.
- Computer Vision:Publicly available generative adversarial networks (GANs) have advanced computer vision applications, enabling the generation of realistic images and videos. These models have been used in areas such as image editing, object detection, and medical imaging.
- Drug Discovery:Generative AI models have been employed in drug discovery research, accelerating the process of identifying and developing new drugs. These models can predict the properties of molecules, generate novel drug candidates, and optimize existing drug structures.
Challenges of Public Generative AI
Public generative AI models, while offering significant benefits, also present a range of challenges that need careful consideration. These challenges are not inherent to the technology itself but rather stem from the way these models are developed, trained, and deployed.
Understanding these challenges is crucial for responsible and ethical use of public generative AI.
Data Privacy and Security Concerns
Public generative AI models are trained on vast datasets that often include personal information. This raises concerns about data privacy and security, as the training data may contain sensitive information that could be exposed or misused. For example, a language model trained on a massive dataset of text and code might inadvertently learn to generate text that reveals personal details, such as names, addresses, or financial information.
This could lead to identity theft, privacy breaches, or other security risks.
The potential for data privacy breaches is a major concern with public generative AI models.
Potential for Misuse and Exploitation
Public generative AI models can be misused or exploited in various ways, leading to harmful consequences. One concern is the potential for generating fake news, propaganda, or malicious content. These models can be used to create realistic-looking images, videos, or text that can be used to deceive or manipulate people.
For example, deepfakes, which are synthetic media that portray real people doing or saying things they never actually did, have become a growing concern.
Deepfakes are a prime example of how public generative AI models can be misused to create harmful content.
Deciding between public or proprietary generative AI is like choosing between a pre-made cocktail and crafting your own. Public AI is like that pre-made drink, accessible and ready to go, while proprietary AI is more like meticulously selecting ingredients and techniques to create something truly unique.
Just like carefully chilling your wines for a New Year’s Eve wine bar, using a DIY ice bucket adds a personal touch, proprietary AI offers greater control and customization, making it a valuable tool for those who want to push the boundaries of what’s possible.
- Another concern is the potential for bias and discrimination. Public generative AI models can reflect and amplify existing biases in the data they are trained on. This can lead to unfair or discriminatory outcomes, particularly in areas like hiring, lending, and criminal justice.
- Public generative AI models can also be used to create harmful or offensive content, such as hate speech or violent imagery. This content can have a significant negative impact on individuals and communities.
Benefits of Proprietary Generative AI: Public Or Proprietary Generative Ai
Proprietary generative AI models, developed and owned by specific companies, offer a unique set of advantages that can be tailored to specific business needs. These models are often trained on exclusive datasets and designed to solve particular problems, providing a competitive edge in various industries.
Specialized Features and Customization Options
Proprietary AI models are known for their flexibility and customization options, allowing businesses to tailor them to their specific requirements. This is a significant advantage over public models, which often have limited customization capabilities.
- Fine-tuning:Proprietary models can be fine-tuned using company-specific data, improving their accuracy and relevance for specific tasks. This process involves adjusting the model’s parameters to enhance its performance on a particular dataset.
- Integration with Existing Systems:Proprietary models are often designed to integrate seamlessly with existing systems and workflows, minimizing disruption and maximizing efficiency. This integration allows for smooth data flow and eliminates the need for complex adaptations.
- Customizable Outputs:Proprietary models can be trained to generate outputs in specific formats or styles, catering to the unique needs of a company. This allows for greater control over the generated content, ensuring it aligns with brand guidelines and internal standards.
Examples of Proprietary AI Models in Specific Industries
Proprietary AI models are being deployed across various industries, revolutionizing processes and driving innovation. Here are a few examples:
- Healthcare:Pharmaceutical companies use proprietary AI models to accelerate drug discovery by analyzing vast amounts of data and identifying potential drug candidates. These models can also be used to personalize treatment plans and predict patient outcomes.
- Finance:Financial institutions utilize proprietary AI models for fraud detection, risk assessment, and algorithmic trading. These models can analyze complex financial data, identify patterns, and make informed decisions, improving efficiency and reducing risk.
- Manufacturing:Manufacturers leverage proprietary AI models for predictive maintenance, optimizing production processes, and improving quality control. These models can analyze sensor data, predict equipment failures, and identify potential bottlenecks in the manufacturing process, leading to increased efficiency and reduced downtime.
Challenges of Proprietary Generative AI
Proprietary generative AI models, while offering unique advantages, also present certain challenges that users and organizations need to consider. These challenges are mainly related to potential risks, limitations, and accessibility concerns.
Vendor Lock-In and Limited Customization, Public or proprietary generative ai
The exclusive nature of proprietary models can lead to vendor lock-in, restricting users to a single provider’s ecosystem. This can limit flexibility and customization options, potentially hindering users from tailoring the AI to their specific needs.
- For instance, if a company relies heavily on a proprietary AI model for its core operations, switching to another provider might require significant effort and resources, potentially disrupting workflows and impacting business continuity.
Cost and Accessibility
Proprietary AI models often involve significant development and maintenance costs, which can translate to higher pricing for users. This can make these models inaccessible to smaller organizations or individuals with limited budgets.
- For example, some advanced proprietary language models, such as those used for natural language processing, might require substantial licensing fees, making them financially prohibitive for many.
Real-World Applications
Generative AI models are rapidly transforming various industries, driving innovation and efficiency. Both public and proprietary models offer unique advantages and are finding widespread use in diverse applications. This section explores real-world examples of how these models are being used, highlighting their key benefits.
Public Generative AI Applications
Public generative AI models, often available through APIs or cloud platforms, provide accessible and versatile tools for developers and businesses. Here are some notable applications:
- Content Creation:Public models like GPT-3 are used for generating high-quality written content, such as articles, blog posts, social media updates, and even marketing copy. These models can help businesses create engaging and informative content at scale, saving time and resources.
- Image Generation:Models like DALL-E 2 and Stable Diffusion are used for creating stunning and realistic images based on text prompts. This has applications in design, advertising, and even the creation of artwork.
- Code Generation:Models like Codex can assist developers in writing code, suggesting solutions, and even generating entire programs. This can significantly improve developer productivity and reduce the risk of errors.
- Translation:Public models are used for translating text between languages, making it easier for businesses to reach global audiences. These models can translate documents, websites, and even spoken language.
Proprietary Generative AI Applications
Proprietary generative AI models are often developed and deployed within organizations to address specific business needs and maintain data security.
Application | Type of AI | Key Benefits |
---|---|---|
Personalized Product Recommendations | Recommender Systems | Improved customer experience, increased sales, and reduced churn |
Fraud Detection | Anomaly Detection | Enhanced security, reduced financial losses, and improved risk management |
Drug Discovery | Molecular Design | Accelerated drug development, reduced costs, and increased success rates |
Personalized Healthcare | Medical Diagnosis and Treatment | Improved patient outcomes, reduced healthcare costs, and personalized care plans |
Future Trends and Developments
The field of generative AI is rapidly evolving, with continuous advancements in algorithms, computational power, and data availability. Both public and proprietary generative AI models are poised for significant growth and transformation in the coming years. This section will explore key trends and developments shaping the future of generative AI and its impact on various industries.
Increased Model Complexity and Scalability
Generative AI models are becoming increasingly complex and scalable, driven by the availability of massive datasets and advancements in deep learning algorithms. This trend is evident in the development of large language models (LLMs) like GPT-3 and PaLM, which possess billions of parameters and can generate human-quality text, translate languages, write different kinds of creative content, and answer your questions in an informative way.
- Advancements in Model Architecture:Researchers are constantly developing new model architectures and techniques to improve the performance and efficiency of generative AI models. For example, transformer-based models, like those used in GPT-3 and BERT, have revolutionized natural language processing and are now being applied to other domains like image and audio generation.
- Increased Data Availability:The exponential growth of data, fueled by the rise of the internet and the Internet of Things (IoT), provides generative AI models with vast amounts of training data. This data abundance enables models to learn more complex patterns and generate more realistic and diverse outputs.
- Enhanced Computational Power:Advancements in hardware, such as GPUs and specialized AI chips, have made it possible to train and run large-scale generative AI models efficiently. This increased computational power allows for the development of more sophisticated models with greater capacity.
Integration with Other Technologies
Generative AI is increasingly being integrated with other technologies, such as robotics, virtual reality (VR), and augmented reality (AR), to create innovative applications.
- Generative AI in Robotics:Generative AI models can be used to train robots to perform complex tasks, such as grasping objects, navigating environments, and interacting with humans. This integration is revolutionizing the field of robotics and enabling the development of more intelligent and adaptable machines.
- Generative AI in VR and AR:Generative AI can be used to create immersive and interactive VR and AR experiences. For example, AI-powered models can generate realistic 3D environments, characters, and objects, enhancing the user experience in virtual worlds.
Personalized and Contextualized AI
Generative AI is moving towards personalization and contextualization, tailoring outputs to individual users and specific situations.
- Personalized Content Generation:Generative AI models can be trained on individual user data to generate personalized content, such as recommendations, news articles, and creative works. This allows for a more customized and relevant user experience.
- Contextualized AI:Generative AI models are being developed to understand and respond to context, enabling them to generate more appropriate and meaningful outputs. This is crucial for applications such as conversational AI, where understanding the context of a conversation is essential for generating relevant responses.