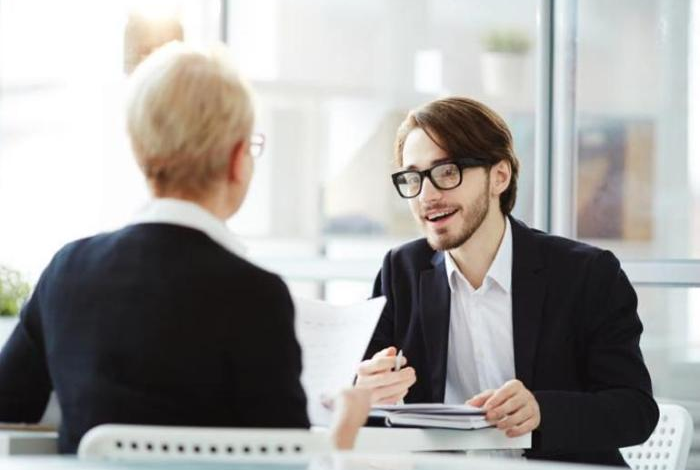
10 Questions Data Scientists Should Ask Employers
10 questions data scientists should ask employers during a job interview – Navigating the job interview process as a data scientist can feel like deciphering a complex algorithm. While showcasing your skills is crucial, it’s equally important to understand the company’s data landscape, culture, and opportunities. Asking insightful questions not only demonstrates your curiosity but also reveals whether the role aligns with your career goals and aspirations.
So, before you accept that offer, arm yourself with these 10 critical questions to ask employers during a data science interview. These questions will help you gain clarity on the team, the data, the projects, and the company’s overall vision, ensuring you make a well-informed decision.
The Opportunities for Innovation
A data scientist’s role goes beyond just analyzing data; it’s about using that data to drive innovation and create tangible business value. This question helps you understand the company’s commitment to data-driven decision-making and its willingness to embrace new technologies.
Areas of Innovation
The company should be able to articulate how data science can contribute to innovation across different areas. For example:
- Product Development:Data science can be used to personalize products, optimize features, and identify new product opportunities.
- Marketing and Sales:Data science can be used to target the right customers, optimize pricing strategies, and improve customer acquisition and retention.
- Operations:Data science can be used to streamline processes, reduce costs, and improve efficiency.
- Customer Experience:Data science can be used to understand customer needs, personalize experiences, and improve customer satisfaction.
Culture of Experimentation and Learning
A company’s culture of experimentation and learning is crucial for data science innovation. This includes:
- Encouraging experimentation:The company should have a culture that encourages experimentation with new data science techniques and approaches.
- Learning from failures:The company should be open to learning from failures and using them to improve future projects.
- Sharing knowledge:The company should have mechanisms for sharing knowledge and best practices across the data science team.
Opportunities for Developing and Implementing New Solutions
The company should provide opportunities for data scientists to develop and implement new data science solutions. This might include:
- Access to resources:The company should provide data scientists with access to the resources they need, such as data, computing power, and tools.
- Support for research and development:The company should support data scientists in conducting research and developing new solutions.
- Opportunities for collaboration:The company should encourage collaboration between data scientists and other teams, such as product, engineering, and marketing.
Examples of Successful Data Science Innovations, 10 questions data scientists should ask employers during a job interview
The company should be able to share examples of successful data science innovations that have had a positive impact on the business. These examples could include:
- Improved product recommendations:A data science team may have developed a new algorithm that improved product recommendations, leading to increased sales.
- Optimized marketing campaigns:A data science team may have used data to optimize marketing campaigns, resulting in higher click-through rates and conversions.
- Reduced operational costs:A data science team may have used data to identify inefficiencies in operations, leading to cost savings.
The Challenges and Opportunities: 10 Questions Data Scientists Should Ask Employers During A Job Interview
Every data science team faces unique challenges, and understanding these challenges is crucial for evaluating a potential employer. A data scientist’s role goes beyond just crunching numbers; it involves problem-solving, collaborating, and driving business impact. This question delves into the specific challenges and opportunities that come with the role, helping you gauge the company’s approach to data science and its commitment to professional development.
Challenges Faced by the Data Science Team
The data science team faces a variety of challenges, ranging from technical hurdles to organizational complexities. These challenges shape the day-to-day work and impact the team’s ability to deliver value.
- Data Quality and Availability:Inconsistent data quality, missing values, and data silos are common challenges. Accessing and cleaning data can consume a significant portion of a data scientist’s time, impacting the speed and accuracy of analysis.
- Scalability and Performance:As data volumes grow, data science models need to be scalable and performant. Handling large datasets and deploying models in a production environment can be complex, requiring specialized infrastructure and expertise.
- Model Interpretability and Explainability:Understanding the reasons behind model predictions is essential for trust and accountability. Complex models, especially deep learning models, can be difficult to interpret, making it challenging to explain their decisions.
- Integration with Business Processes:Successfully implementing data science solutions often requires seamless integration with existing business processes. This can involve overcoming resistance to change, aligning data science outputs with business objectives, and ensuring effective communication between data scientists and stakeholders.
Overcoming Challenges
Companies have various strategies for addressing these challenges. Understanding the company’s approach provides insight into its commitment to data science and its ability to support its team.
- Data Governance and Management:Implementing robust data governance policies and investing in data management tools can improve data quality and availability. This includes establishing data standards, implementing data quality checks, and providing access to reliable data sources.
- Infrastructure and Tools:Companies often invest in cloud computing platforms, distributed computing frameworks, and specialized data science tools to enhance scalability and performance. This allows data scientists to work with large datasets efficiently and deploy models in production environments.
- Model Interpretability and Explainability:Companies are increasingly adopting techniques and tools to enhance model interpretability and explainability. This includes using simpler models, feature importance analysis, and visualization techniques to provide insights into model behavior.
- Collaboration and Communication:Fostering strong collaboration between data scientists, domain experts, and business stakeholders is crucial for successful implementation. This can involve establishing clear communication channels, defining roles and responsibilities, and promoting a culture of knowledge sharing.
Opportunities for Personal and Professional Growth
A data science role offers opportunities for continuous learning and professional development.
- Exposure to New Technologies:The rapidly evolving field of data science exposes you to new technologies, algorithms, and tools. This allows you to stay ahead of the curve and expand your skillset.
- Solving Complex Problems:Data science projects often involve tackling complex and challenging problems. This provides an opportunity to develop critical thinking, problem-solving, and analytical skills.
- Collaboration and Communication:Working on data science projects involves collaborating with diverse teams and communicating technical concepts to non-technical stakeholders. This enhances your communication and teamwork skills.
- Impacting Business Outcomes:Data science has the potential to drive significant business value. Witnessing the impact of your work can be incredibly rewarding and motivates continuous improvement.
Company Support for Data Scientists
Companies that prioritize data science often provide dedicated support for their data scientists.
- Training and Development Programs:Companies may offer training programs, workshops, and mentorship opportunities to help data scientists stay up-to-date with the latest advancements and develop their skills.
- Access to Resources:Providing access to cutting-edge technology, tools, and data resources is essential for data scientists to perform their work effectively.
- Recognition and Rewards:Companies should recognize and reward data scientists’ contributions, creating a motivating and supportive environment. This can involve performance bonuses, promotions, and opportunities for leadership development.
- Culture of Innovation:A company culture that encourages experimentation, collaboration, and learning is essential for data science to thrive. This allows data scientists to explore new ideas, take risks, and contribute to innovation.
When interviewing for a data science role, it’s crucial to understand the company’s data landscape and how you’ll contribute. Asking about their approach to user acquisition, for example, can reveal valuable insights. Do they leverage app store search ads to drive app downloads?
This can tell you about their marketing strategies and the potential impact of your work on user growth. By asking these targeted questions, you can gauge the company’s data maturity and determine if it aligns with your career goals.
Before diving into the nitty-gritty of data science projects, it’s crucial to understand the bigger picture. Asking questions about the company’s data infrastructure, data quality, and team dynamics can shed light on the potential challenges and opportunities. For example, you might inquire about the tools and technologies used, similar to how I was curious about the design choices behind elsies breakfast nook before.
Understanding these aspects can help you assess if the role aligns with your skills and interests, ultimately leading to a more fulfilling and successful career in data science.
When interviewing for a data science role, it’s crucial to ask questions that reveal the company’s data culture and your potential impact. Just like I learned the importance of understanding soil composition and sunlight exposure in what ive learned so far from my first garden , asking questions about data infrastructure, project timelines, and collaboration styles can help you determine if the role is the right fit for you.
Remember, a successful data scientist needs the right environment to thrive, and insightful questions can help you find it.